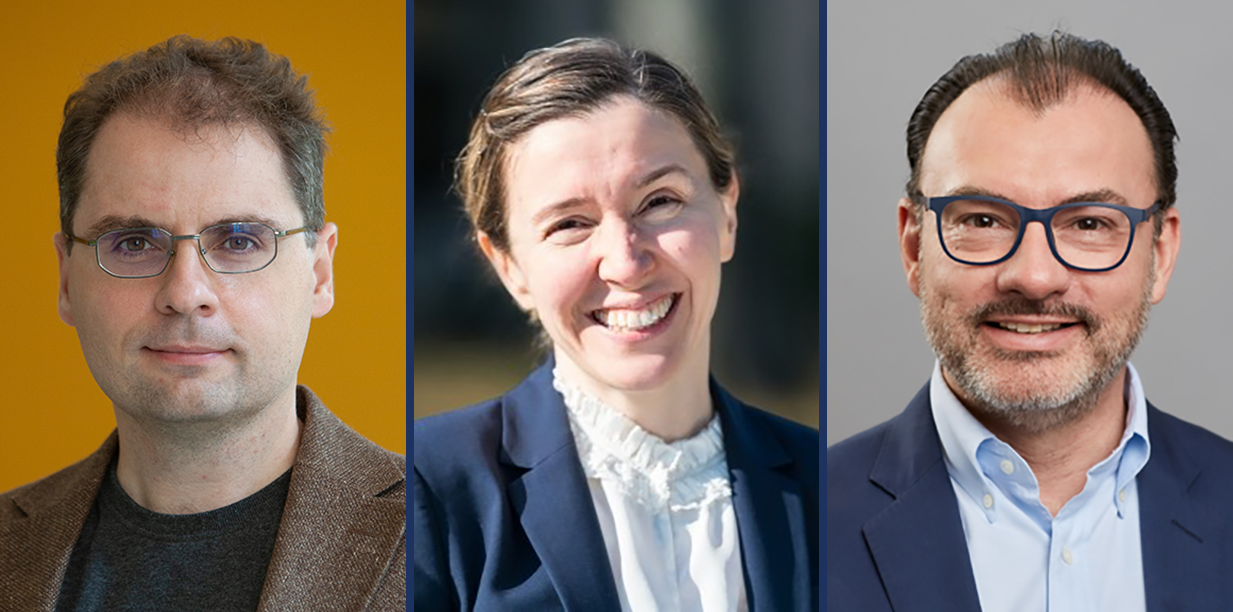
The AI Policy Forum is an undertaking by the MIT Schwarzman College of Computing to bring together leaders in government, industry, academia, and civil society from around the world to develop approaches to deal with the societal challenges posed by the rapid advances and increasing applicability of artificial intelligence. Formed in late 2020, the overarching goal of the AI Policy Forum is to bridge high-level principles of AI policy with the practice and tradeoffs of governing.
As part of this global effort, the AI Policy Forum is convening members of the public sector, private sector, and academia for a virtual event to discuss critical questions in AI policy. Taking place Thursday, May 19 from 9:00 am to 4:00 pm EDT, the second AI Policy Forum Symposium will feature an array of panelists covering cross-sector topics such as the design of AI laws and AI auditing, as well as sector-specific issues like AI in healthcare and mobility.
Co-leading the AI Policy Forum are Aleksander Madry, the Cadence Design Systems Professor; Asu Ozdaglar, deputy dean of academics for the MIT Schwarzman College of Computing and head of the Department of Electrical Engineering and Computer Science; and Luis Videgaray, director of MIT AI Policy for the World Project.
They discuss here some of the issues that will be explored during the symposium, including the impact of AI across sectors, trends in AI technology, and why we still need to build understanding and practice of effective AI policymaking.
Q: How has the AI policy landscape been evolving in recent years?
Videgaray: After a long period of silence, recent years have brought a real flurry of activity around the development of AI laws—from the United States passing the National Artificial Intelligence Initiative Act, to Europe having passed several laws and moving forward its own EU AI Act, to even China advancing several new regulations on AI. However, these legislative efforts tend to follow different schools of thought, which makes the discussion shift towards the question: what makes an AI law a “good” law?
Of course, what constitutes a “good” AI law might be highly subjective and context-dependent, but creating an AI law still inevitably involves making certain key design choices. One of them is whether the legislation should establish binding rules or constitute more of a voluntary guideline. Another one is whether the regulations should be horizontal, i.e., cross-sectoral, or vertical, i.e., domain-specific. Yet another consideration is whether the focus should be on regulating the inputs to the AI systems, i.e., the training data, or more on controlling their outputs (akin to the disparate impact doctrine in consumer finance).
All these aspects—and their relative merits and disadvantage—are currently hotly debated. We still need to build our understanding and practice of effective AI policymaking.
Q: What trends do you see in AI technology that can have an immediate impact on the policy space?
Madry: AI is a very rapidly evolving technology so there could be a number of trends to discuss. Still, one trend I find particularly worth highlighting is the evolution of how machine learning is currently developed and deployed. Specifically, it increasingly becomes the case that the organizations utilizing machine learning outsource not only the creation of data used to train their machine learning models but even the very training of these models. This results in a progressing vertical segmentation of the AI supply chain and, more broadly, the prevalence of the AI-as-a-Service model. In fact, machine learning service delivery starts to constitute a chain of multiple businesses that ultimately connect to the end consumer.
These developments have major implications for AI policy. For example, how to address the corresponding fragmentation of responsibility? Or how to tackle the risks that might arise at the “seams”? What about the fact that the market concentration might give rise to “mono-cultures” that could give rise to instability or systemic discrimination?
All of these challenges are exacerbated by the recent emergence of very large “core” or “foundation” models, such as Google’s BERT, OpenAI’s GPT-3, Huawei’s PanGu, BAAI’s Wu Dao or Meta’s OPT-175B. The policy implications of this emergence are bound to be a topic of much discussions between technologists and policymakers alike.
Q: What sectors require the most urgent attention from the AI policy perspective?
Ozdaglar: Many sectors are currently disrupted by AI. However, the three sectors I would single out as undergoing fundamental transformation and requiring much more attention from an AI policy point of view are: healthcare, mobility, and social media.
For healthcare, the challenge is how to regulate the deployment of AI-driven solutions. For example: how should we strive to combine safety, equity, and performance considerations? Sharing of medical data is critical for performance but creates a whole slew of issues related to safety and equity, especially given the sensitive nature of these data and how they can be misused. Dealing with these issues requires both regulation and the design of new technologies that can leverage the benefits of data, while respecting and protecting privacy.
In the context of mobility, partially autonomous driving is already a reality. It is unclear, however, whether fully autonomous driving will be feasible in the near future, and how its safety can be guaranteed. There are also equity issues in mobility: Who will benefit most from deployment of self-driving technology? What will be the impact on the millions of people working in the transport industry such as truck drivers and delivery workers? On those relying on public transport? What innovations do we need in infrastructure?
Finally, in the context of social media, the prevalence of misinformation, hate speech, and broader mental health issues associated with social media are critical questions. There is growing evidence that many of these are related to the algorithmic choices social media platforms are making. For example, misinformation seems to spread much more virally in filter bubbles created by such algorithms. This raises questions related to regulation and business models of social media platforms. There was a great deal of promise from the democratization of communication. The question is whether any of that promise can be realized, or whether we should just attempt to limit the detrimental effects of social media.
For more details on the AI Policy Forum Symposium and to register, visit the event page.